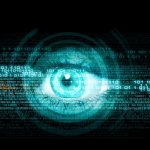
AIOps – Beyond the Hype
May 7, 2021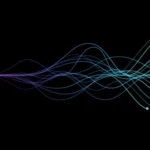
AIOps from Ennetix – Built to Last on a Strong Foundation
June 7, 2021Digital transformation, being widely embraced by enterprises today, has many economic benefits; however, it is also causing the enterprise’s IT operations to become increasingly chaotic. To reduce this chaos and create determinism in digital operations, e.g., for faster and accurate resolution when problems arise, AIOps (Artificial Intelligence for IT Operations) can play a very important role.
In the digital transformation journey, enterprises of all sizes are increasingly adopting various novel paradigms and platforms such as public cloud, hybrid cloud, multi-cloud, virtualized services, third-party apps, microservices, serverless computing, virtual desktops, work-from-home technologies, etc., to build and support their mission-critical applications for continued business success.
As a result, the enterprise’s application-delivery infrastructures are becoming more virtual, distributed (across the Internet) as well as dynamic (i.e., an application’s infrastructure resources could vary in size, location, and time of the day, depending on user demands). These resources come and go (known as “resource churn”) based on demands; same is true about the users of these applications – their location, type, and demand vary all the time
In such a dynamic, virtualized, and chaotic world, creating order to achieve sustained and premium digital operations is of utmost importance.To manage such a distributed and dynamic environment, the enterprise’s IT operations teams rely on an increasingly wide variety of logs/metrics and tools for performance monitoring, analytics, etc.
The volume, velocity, and variety of these collected metrics/logs are also increasing rapidly. To ingest and make sense out of this “big data” and automate parts of the IT operations processes, a major role is being played by Artificial Intelligence (AI) techniques such as Machine Learning (ML), leading to the term AIOps. Some specific examples of AIOps solutions include: correlating “events” (from multiple sources); detecting anomalies; etc.
Now, if we consider an enterprise’s digital operations supporting business-critical applications, there are three dimensions to it: applications, infrastructure resources (supporting the applications), and users (of the applications). As mentioned before, resources and users are dynamic (exhibiting churn), so the only thing that is deterministic in these enterprise IT environments is the “applications” (and their supported business goals).
Therefore, to achieve determinism in digital operations, AIOps solutions should provide and uplift their results and analytics tied to the business-critical applications. This leads to a paradigm, called “Application-Centric” AIOps, where applications and their causal relationships with resources and users can be discovered and used to effectively provide deterministic and application-oriented outcomes in digital operations AI/ML-powered algorithms (e.g., anomaly detection) are inherently based on probabilistic analyses.
Like all probabilistic models, these solutions also tend to provide false alarms – (1) false positives (viz. stating there is a problem when there isn’t one) and (2) false negatives (viz. not being able to find the problem when there is one). How can an AIOps solution reduce these false alarms and improve determinism in digital operations? One way to improve the accuracy of these AI/ML methods is to cross-validate events across multiple sources.
AIOps solutions should seek to collect, analyze, and correlate (using their causal relationships) multiple and encompassing sources of relevant data, and highlight the ground truth in those data sets. This means that, even if an enterprise employs separate tools for application performance monitoring (APM), network performance monitoring (NPM), event log collection, log analytics, etc., it still needs an overarching AIOps solution that can analyze and correlate the chaotic set of events generated by multiple sources from their own “silo-ed” perspective, and create accuracy, i.e., determinism, by reinforcing events and minimizing false alarms.
The more data an AIOps solution can collect (or has access to), analyze, and correlate, the better are the chances to reduce the false alarms and hence improve the visibility/observability gap in the IT infrastructure. This process will ultimately improve the efficacy of the AIOps solution, reduce the probabilistic outcome of the process, and uptick the accuracy and determinism of the enterprise’s digital operations!