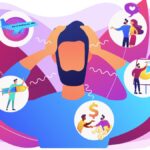
TikTok and the surveillance economy
March 26, 2024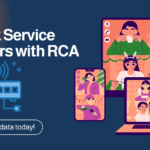
Enhancing ISP Network Reliability Through Effective Root-Cause Analysis
June 11, 2024Root-cause analysis (RCA) is a problem-solving method that aims to identify and address the underlying causes of a problem or issue, rather than just treating the symptoms or applying quick fixes. This approach helps organizations in various industries to improve their processes, minimize errors, increase efficiency, and prevent recurring issues. Root-cause analysis is particularly important in industries such as enterprises, retail, manufacturing, government, and academia, where even a minor issue can have significant consequences.
Key Objectives of Performing RCA
- Identify the primary causes of an issue,
- Understand why these causes occurred, and
- Develop effective solutions to prevent similar problems in the future.
The Increasing Complexity of Automated Root cause Analysis
The increase in data volumes and the variety of data types, especially in today’s cloud-centric virtualized IT infrastructures, make RCA a complex task. Traditional methods struggle to analyze and make sense of the vast amounts of infrastructure data, especially in real time. The inability to effectively correlate events and automate event correlations further compounds the problem. Consequently, the RCA process often becomes time consuming and inefficient; and it provides insufficient actionable insights. The result is prolonged Mean Time to Acknowledge (MTTA) and Mean Time to Repair (MTTR), leading to compromised application performance, user experience, and overall business outcomes.
The Impact on Performance and Business Outcomes
The limitations of traditional RCA methods have a direct impact on application and user performance, as well as business outcomes. High MTTA and MTTR result in delays in identifying and addressing performance issues, leading to prolonged system disruptions and dissatisfied users. The lack of actionable data in the RCA process prevents organizations from taking fast and automated remediation actions, further exacerbating the problems.
Streamlining RCA with Advanced Technologies
To overcome these challenges, organizations need a new approach to RCA that can effectively handle the increasing complexity of data in today’s cloud-centric virtualized IT infrastructures,
and accelerate the identification and resolution of issues. Advanced technologies, such as Artificial Intelligence (AI) and Machine Learning (ML), play a crucial role in simplifying and automating the RCA process. Some advanced technologies that are being utilized in streamlining RCA are the following:
- Data-driven RCA: By leveraging AI and ML algorithms, organizations can analyze large volumes of heterogeneous infrastructure data more efficiently. These technologies can identify patterns, anomalies, and correlations in real time, enabling faster and more
accurate root-cause identification. - Automated Event Correlation: AI-powered RCA solutions can automate the correlation
of IT events, reducing manual efforts and providing a comprehensive overview of the infrastructure. Organizations can prioritize their response and remediation efforts by identifying causal relationships between events. - Actionable Insights: With AI-driven RCA, organizations can extract actionable insights
from the vast amount of collected data. These insights enable IT teams to take proactive
remediation actions, such as automated or guided remediation, before performance
issues impact applications and user experiences. - Reduced MTTA and MTTR: By streamlining the RCA process with advanced
technologies, organizations can significantly reduce MTTA and MTTR. Rapid identification and resolution of issues lead to improved application performance, enhanced user satisfaction, and better overall business outcomes.
AI and ML technologies are revolutionizing RCA, enabling organizations to automate and enhance the process. These technologies can analyze vast amounts of data, identify patterns and correlations, and generate insights that may not be readily apparent to human analysis. Automated RCA systems can quickly identify the root causes of problems, saving organizations valuable time and resources.
What is Automated Root-Cause Analysis?
Automated Root-Cause Analysis is a technology-driven approach designed to identify the primary reasons behind anomalies, incidents, or failures in IT systems and networks. Unlike traditional RCA, which relies heavily on manual intervention and expertise, automated RCA utilizes sophisticated algorithms, typically powered by Artificial Intelligence (AI) and Machine Learning (ML), to analyze vast amounts of data quickly and efficiently.
Steps to Achieve Automated RCA
- Data Collection: Automated systems continuously monitor and collect data from various sources within IT infrastructures, including logs, metrics, and performance indicators.
- Anomaly Detection: AI algorithms are trained to recognize patterns and deviations in the data that represent potential issues. These anomalies could range from minor irregularities to indicators of significant failures.
- Root-Cause Identification: Once an anomaly is detected, the system then uses Machine-Learning (ML) models to sift through the huge amount of collected data, identifying correlations and causations that point to the underlying root cause of the anomaly.
- Actionable Insights: The final step involves presenting the findings comprehensibly. The system may suggest potential fixes, alert relevant personnel, or initiate automated corrective actions in some advanced setups.
The main advantage of automated RCA is its ability to reduce the Mean Time to Acknowledge (MTTA) and Mean Time to Repair (MTTR) significantly. By automating the detection and analysis process, organizations can proactively address issues before they escalate into critical failures, minimizing downtime and potential revenue loss.
xVisor by Ennetix exemplifies this new era of IT operations management, emphasizing the prevention of problems rather than just reacting to them. This proactive approach is made possible by harnessing the predictive power of AI and ML, marking a significant evolution in how businesses efficiently manage and maintain their IT infrastructure.